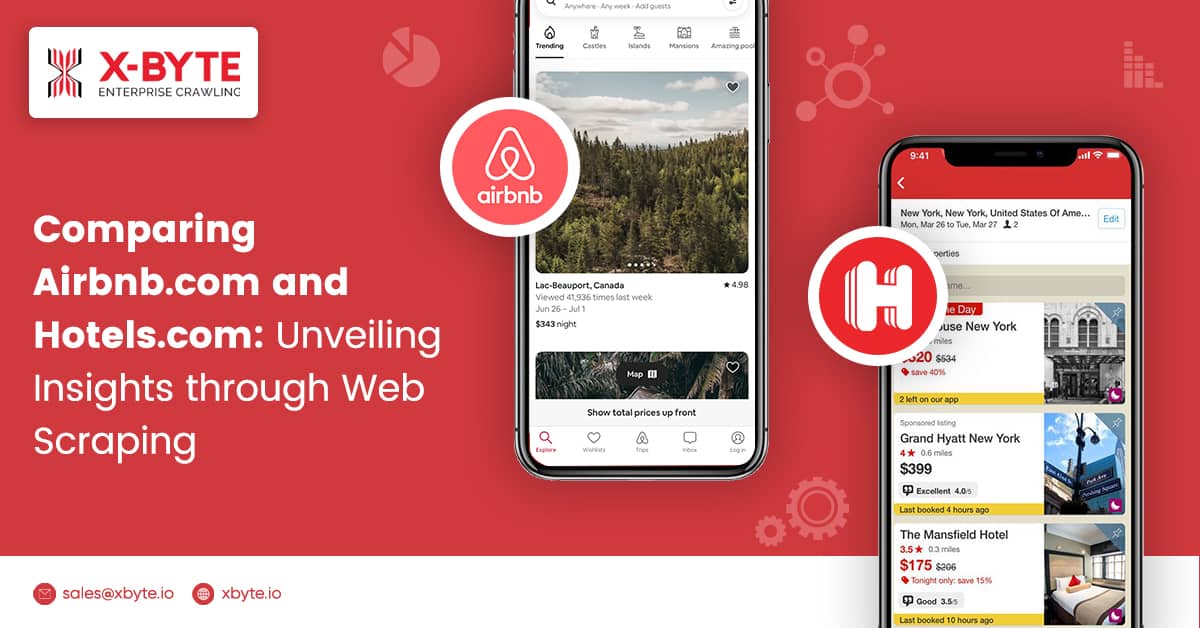
We frequently need help with the decision of where to stay while traveling. The possibilities were few earlier, but now, thanks to the booming hotel industry, we have a wide range of choices. We may reserve hotel rooms online well in advance after carefully comparing numerous criteria, including price and facilities. We may choose the best solution after evaluating the available choices. These days, a few websites take care of this for us and streamline the procedure. They gather information from many hotel websites and facilitate our ideal accommodation choice. Numerous systems provide data scraping, a kind of data collection. The two most prominent platforms are Airbnb.com and Hotels.com. This article will stress a comparative analysis of these sites based on their advantages.
Background Study
Before delving into the comparative analysis of Web Scraping Airbnb and Hotels, we need to focus on the background story of the emergence of these two portals.
Airbnb.com came into being in 2008 in San Francisco in the form of a community-based web platform for selling and renting local homes. It links hosts and travelers and facilitates the rental process, although it owns no dwellings. It also promotes a shared economy by allowing housing owners to lease units in their own right. It generates money by charging visitors a 6-12% non-refundable service fee. Again, in the case of the hosts, it charges a 3% processing fee.
On the other hand, Hotels.com founded in 1991 in Dallas, Texas, is an internet site to book hotel rooms online and over the phone. There are hotel rooms, bed & breakfasts, some apartments, and business establishments in its inventory. It’s a business model based on commissions.
Advantages of Data Scraping
The main aim of web scraping Airbnb and hotels is to acquire a significant amount of data from the targeted websites to present the data to potential clients(travelers) to make selecting the hotels easier for them. Websites like Airbnb.com and Hotels.com are numerous hotels and homestay listings that provide millions of travelers with the necessary insight into the amenities the hospitality industry promises.
Data scraping also allows travelers to learn about the trending locations for planning their next trip based on the data collected from these popular sites like Airbnb.com and Hotels.com. These also highlight the tariffs of these well-known hotels, booking methods, cancellation rules, and the facilities available.
Searching through these listings on travel websites might take a long time to attain key information. However, a programmed web scraping technique offers a creative alternative to acquiring data from thousands of hotel and homestay entries. Thus, travelers can make sound decisions according to their budget and expectations of amenities after comparing varied options.
Relevance of Web Scraping Airbnb and Hotels
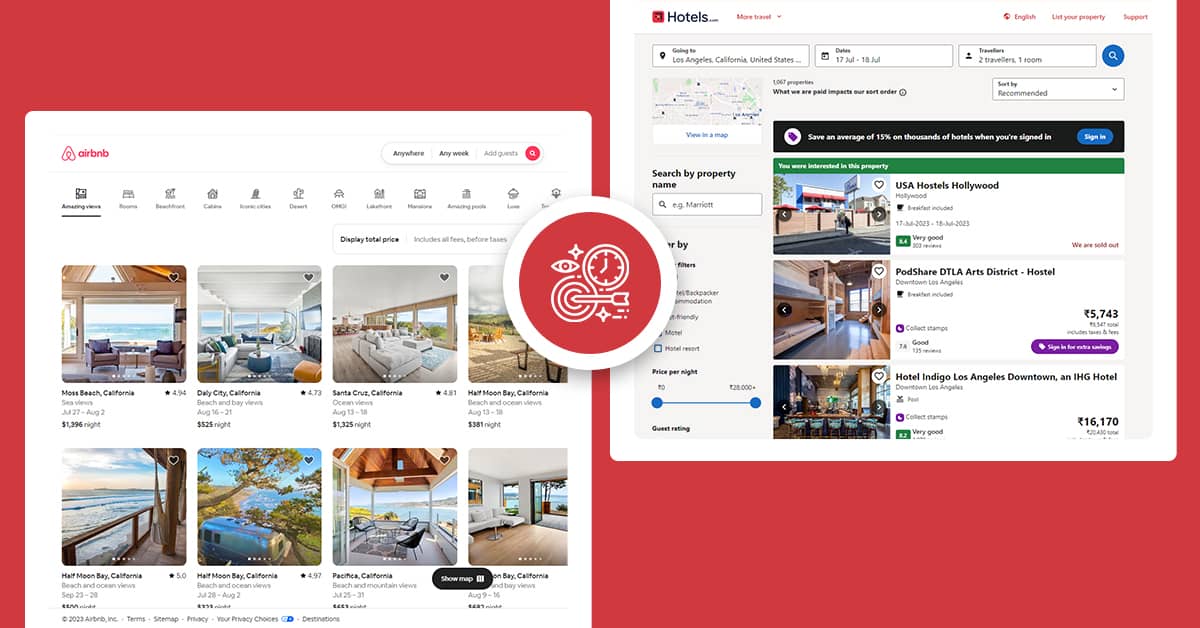
Airbnb.com
For crawling a website like Airbnb, it is advisable to resort to Selenium since AJAX loads the data on this website. Despite the slow speed, the task is performed flawlessly. Since this platform necessitates the user to enter a specific travel location to gather the data, the scraping process becomes time-consuming. Again, it limits the search option to seventeen pages at a time, and on clicking “next” after the seventeenth page, the user is redirected to the first page. No data available on the first seventeen pages are easily accessible using Selenium. Another area for improvement while scraping data in Airbnb is dealing with the layout. In some pages, anomalies occur, which necessitate the use of alternative paths in Selenium. Scrapy Splash is yet another potential choice for attaining data from this website.
Hotels.com
The data scraping process using Selenium for Hotels.com also experiences certain speed and page layout challenges. However, the prominent issue is the inability of the user to explore the listings by clicking on the “next” button. This minor hurdle can be resolved by resorting to the window.scrollTo() method. It entails scrolling to load the listings.
Cleaning and Modification of Data
Sometimes it is necessary to manipulate the data for easy analysis while web scraping Airbnb and hotels. For instance, for statistical analysis, only numerical values are needed. So according to this requirement, we can clean the data or omit certain factors like the currency sign ($) from the tariffs and other factors for data comparison and analysis. Digits are also considered to compare the apartments’ size or number of bedrooms. A pandas data frame may be used to load data from a CSV file and clean it in Python. In the case of Hotels.com, data cleaning, and manipulation may be done using the Python re. Find all () function to attain integers for statistical comparison.
Analysis and Visualization of Data
Data analysis depends on the following important variables:
- The factors influencing tariffs in AirBnb.com and Hotels.com
- Business comparison of both the platforms
- Role of the location in the alteration of prices
- Number of travelers
For this data analysis, seaborn and matplotlib (with ggplot style) may be used for the sample test. It can be used to scrap the data on the price ranges of the different hotels based on different locations. Other factors that may be considered are probability density function, population, and property choice and popularity according to the prices.
Web scraping Airbnb and hotels gives access to listings with reviews that show the choice of accommodation according to popularity amongst reviewers. It is evident in both the platforms of Airbnb.com and Hotels.com.The price of the hotel is also related to the size of the place and the availability of private rooms, the capability of the number of people it may accommodate, ratings given by customers, satisfaction level of customers. Scrapping allows establishing a linear relationship between these factors to reach a decision.
The statistical analysis allows travelers to decide on hotel selection according to the number of guests and the trip budget. For instance, in case of a limited budget, a large group can rent an entire home, while for small groups, average-rated hotels may be a choice. Thus, the analysis helps to attain hospitality with cost-effectiveness.
Practical Utilization of Data Scraping
The ones starting travel websites benefit the most through web scraping Airbnb and hotels by collecting the relevant data and presenting it user-friendly on their platforms. They can focus on listing according to competitive prices and potential customers’ preferences. Thus, it helps them formulate promising marketing strategies. Finally, they get an insight into the market overview and can devise the perfect way of converting visitors to their websites.
Comparative Study
Data scraping on both platforms depict specific trends. After crawling the websites, it becomes evident that youngsters/millennials in today’s age prefer Airbnb while the ones traveling with family opt for booking from Hotels.com. Again, data also defines that the price of an Airbnb listing is significantly related to the size of the property and the number of guests. However, the popularity of a place does not enhance the prices, and hence top-rated places are not necessarily expensive.
On the other hand, bookings through Hotels.com define a linear relationship between ratings and prices. The top-rated hotels are more costly than high-rated Airbnb, and the review of the customers depicts greater positive aspects in the case of Airbnb than in hotels. Nonetheless, it also brings to the forefront that specific Airbnb rentals are cheaper than hotels, but those confer poor services.
Conclusion
The idea of web scraping to get information on lodging possibilities via Airbnb.com and Hotels.com sheds light on several critical considerations for travelers. For instance, evidence indicates that Airbnb still needs to offer the same level of protection that hotels do. Additionally, there are more facilities available in hotels. As a result, thanks to web crawling, the data allows for comparative analysis, and the decision is easy for travelers.