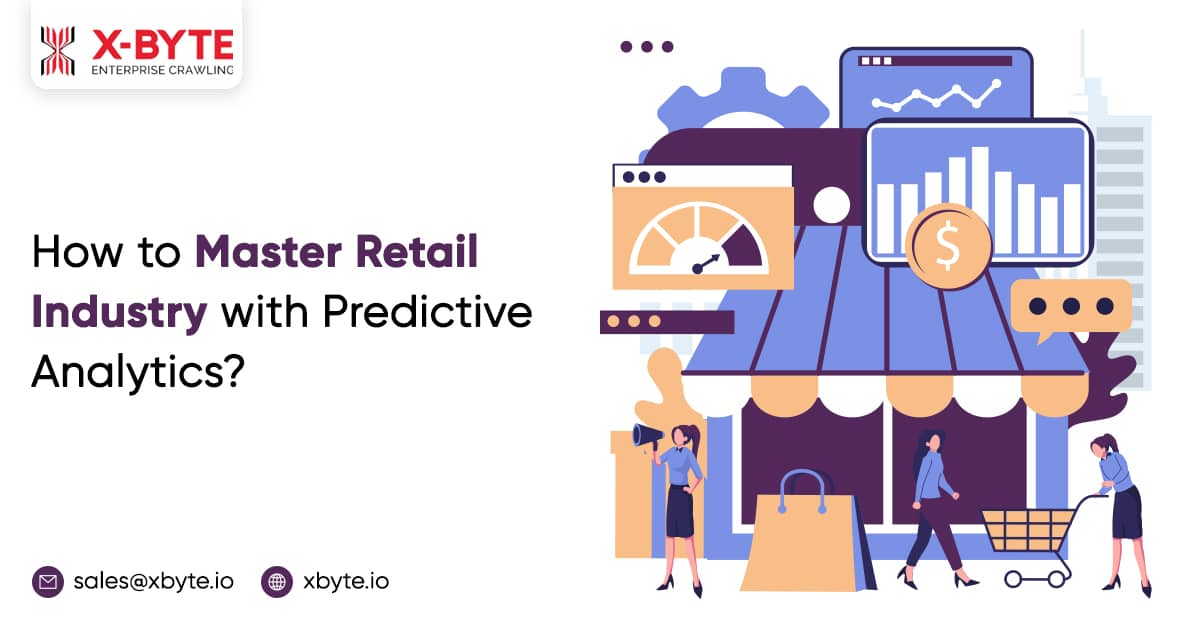
Today, shoppers have more options than ever, and what they want keeps changing. Retail stores must stay ahead of these changes in this dynamic market. Predictive analytics is an intelligent way for retail data analytics to get ahead by guessing what customers want and noticing trends ahead of time. It looks closely at data, like what people have bought before and what’s trending on social media, to predict what shoppers might do next. This blog will be your easy-to-follow guide to how predictive analytics is used in the retail industry. We’ll see how it can help make shopping experiences more personal, smooth supply chain operations, and help stores stay profitable. By looking at new technologies and the best practices, we’ll see how predictive analytics in retail is not just changing the game but creating a whole new game. This helps stores not only meet but go beyond what their shoppers expect.
Understanding Predictive Analysis in Retail
Predictive Analysis in Retail is a way to guess what shoppers might do next. It uses past purchases, habits, likes, what’s popular in the market, and other essential details to predict how shoppers might behave, what they might want to buy next, and how to improve the store’s day-to-day operations.
Here’s a breakdown of the critical components involved:
- Data Collection: Predictive analytics starts with collecting many different types of information. This includes details about the customers (like age or location), what they’ve bought before, how they browse for products online, data from reward programs, their social media interactions, and even weather patterns, depending on what kind of business you run.
- Data Mining: After gathering all the data, the next step is to find useful information hidden in it. This is done using data mining techniques to spot patterns, connections, and hidden patterns in large amounts of data.
- Modeling: Based on the extracted insights, statistical models are built. These models can predict future sales, customer churn, product popularity, and other vital metrics.
- Machine Learning: Advanced technologies, which are part of machine learning, can make predicting things easier and refine predictions as they have more new information to work with.
Benefits of Predictive Analysis in Retail
Predictive analytics benefits store owners, helping them make choices based on data and improving how they run their businesses. Here are a few advantages:
01. Enhanced Customer Experience
Predictive Analytics uses Retail Data Analytics to create a unique shopping experience for each customer. By guessing what customers might like, store owners can suggest products and deals that match their tastes, making customers happier and more likely to stick around.
02. Improved Inventory Management
Data Analytics for Retailers helps predict exactly what customers will want, reducing the need for too much or too little stock. This means stores will have just the right items when needed, making the best use of money and shelf space.
03. Targeted Marketing
Retail Data Analytics lets stores segment customers and what they like to buy. This information helps create tailored marketing plans, which leads to more sales and a better return on the money spent on advertising.
04. Dynamic Pricing Strategy
Predictive Analytics in Retail can predict which products will be popular, allowing stores to change prices as needed. This means they can lower or raise prices at just the right time based on what’s in demand to sell more and make more money.
05. Risk Management
Data Analytics for Retailers helps spot possible problems, like fraud or issues related to the supply chain. This allows stores to act early to avoid these problems, making things run more smoothly and keeping profits safe.
Basic Models of Predictive Analytics in Retail
The predictive analysis models act as building models to develop complex statistical algorithms for more applications. Here are the basic models:
Regressive Model
This is a way of using statistics to find connections between different variables. In retail, it can help guess future sales using old sales records, how changing prices will affect how much people want to buy, or how special deals can change what customers do.
Market Basket Analysis (MBA)
This method looks at what items people often buy simultaneously – like noticing that peanut butter and jelly are often bought together. By spotting these trends, stores can recommend products that go well together to customers, which might help them sell more.
Churn Prediction Models
These models guess which shoppers might stop buying from a store. This information helps stores determine what they can do to keep these people shopping with them.
Customer Lifetime Value Models
This estimates the total value a customer will bring to a retailer over their relationship. This helps focus efforts on the most valuable customers.
Classification Models
These models sort information into specific groups. Stores might use this to put customers into different groups based on what they buy, who they are, or their browsing history. This makes creating ads for certain people or suggesting products they might like easier.
Emerging Trends in Predictive Analysis in Retail
Predictive analysis is evolving, and some new trends are beginning to change its future. Let’s explore some of these emerging trends in predictive analysis.
Augmented Reality (AR) and Virtual Reality (VR)
The use of artificial intelligence and machine learning automates jobs and makes it easier for everyone to get useful information. Imagine a user-friendly interface using natural programming language to help those who aren’t experts make sense of complicated information and make predictions.
Real-time Analytics
Stores are using smart devices and stronger computing systems to analyze data immediately, which helps them make quick business choices, like changing prices as needed or offering special deals just for you.
Sustainability and Ethical Sourcing
Consumers are increasingly concerned about sustainability and ethical practices. Predictive analytics is being used to forecast consumer demand for sustainable products, optimize supply chains for reduced waste, and ensure ethical sourcing.
Voice and Image Search
More people are using voice commands and picture searches to find what they want to buy. Stores are using recognition technology to improve their performance in these new search methods, making sure their websites and products match well with what people can find by speaking or using images.
Explainable AI (XAI)
As artificial intelligence becomes more complicated, it’s important to make it easier to understand. Explainable AI, or XAI, methods help people see how these AI systems make their predictions. This builds trust and helps people make good choices. This is very important in stores for explaining why certain marketing plans or product suggestions based on AI guesses are chosen.
Blockchain for Customer Insights
People are looking into using blockchain, a safe and transparent way of gathering information about what customers do at different shopping points. This could give fresh and useful ideas for predicting what customers will want or do in stores using predictive analysis.
Best Practices for Data Analytics for Retailers
Here are some best practices for retailers when it comes to data analytics:
- Be transparent with customers about what data you collect and how it will be used.
- Obtain customer consent where required and ensure data privacy laws are followed.
- Combine data from different sources (online, in-store, mobile) for a complete view.
- Analyze customer feedback and sales data to find ways to improve. Use chatbots or other AI tools to provide quick assistance.
- Use real-time information to make quick decisions on pricing or promotions.
- Use data to identify the effectiveness of your marketing campaigns and adjust strategies accordingly.
- Protect your data from breaches with good cybersecurity practices. Ensure your team has the necessary knowledge to use analytics tools effectively.
- Stay updated with regulations and any changes that might affect your use of customer data.
Conclusion
In conclusion, predictive retail analysis transforms how businesses understand customers, manage inventory, and personalize marketing efforts. By leveraging historical data, retailers can accurately predict future trends, customer needs, and potential market changes. This optimizes operations and enhances the shopping experience, driving loyalty and sales. However, the backbone of successful predictive analysis is high-quality data. That’s where X-byte comes in. X-byte is a data analytics services provider that provides well-structured and high-quality data from multiple sources, which is important for making accurate predictions. By partnering with X-byte, retailers can ensure they have the reliable data needed to harness the full potential of predictive analytics.